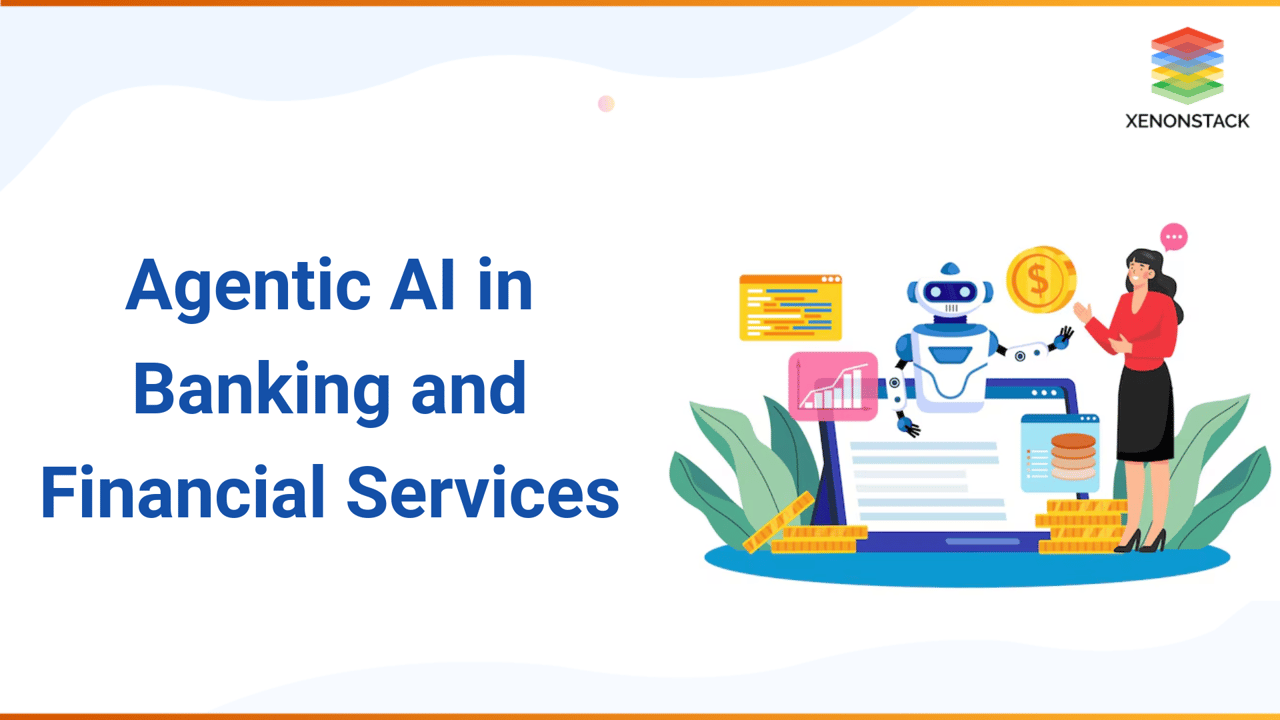
The banking sector has a long history of adapting to technological changes, from traditional automation to Robotic Process Automation (RPA) and now to Generative AI and Agentic AI systems. These sophisticated machine-learning technologies excel at analyzing extensive data sets, allowing financial institutions to produce unique insights and content.
Implementing AI initiatives requires careful strategic planning, talent acquisition, skills development, and effective risk management and change management practices.
The Evolution of AI in Banking
From RPA to Agentic AI
Robotic Process Automation (RPA) in banking, often called accounting process automation, involves using tools such as UiPath, Blue Prism, and Automation Anywhere to reduce the human effort required for processing financial transactions and accounting tasks. These RPA tools help automate data movement between accounting systems and external applications, minimizing manual intervention and improving efficiency.
Instead of thinking of accounting robots as replacements for human workers, RPA has been more akin to a "bionic arm" that enhances the work of finance and accounting professionals. By automating routine data transfer tasks, RPA bots allow workers to focus on higher-value activities, speeding up processes and reducing errors in financial operations.
Today, financial institutions are moving beyond simple RPA to embrace Agentic AI systems, which can automate tasks and make decisions, generate content, and interact with customers more naturally.
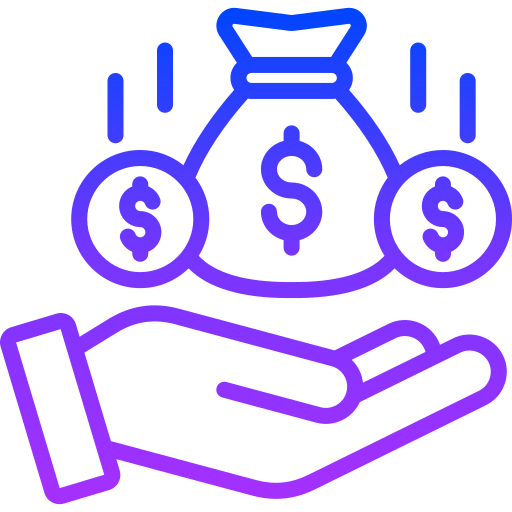
Rapid Market Growth
The global Agentic AI market in banking and finance achieved a valuation of USD 712.4 million in 2023, showcasing significant adoption across the sector
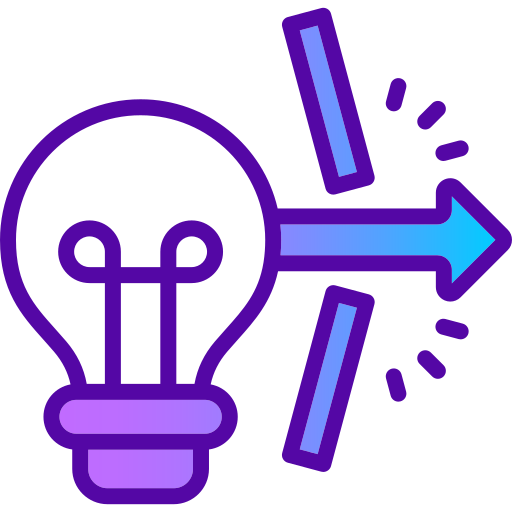
Forecasted Expansion by 2032
Projected to grow at a CAGR of 33%, the market is expected to reach an impressive USD 12,337.87 million by 2032, indicating a transformative impact on financial services
What Does Agentic AI Mean to Banking?
Agentic AI in banking refers to autonomous, goal-driven AI systems that can perceive, reason, decide, and act on behalf of users or institutions to achieve specific financial objectives. Unlike traditional AI models that require predefined inputs and outputs, Agentic AI systems operate with greater autonomy, enabling them to:
-
Initiate and manage complex financial workflows with minimal human intervention
-
Continuously learn and adapt based on real-time data, customer behaviour, and regulatory shifts
-
Make context-aware decisions to optimize operations across customer service, compliance, risk management, and fraud detection.
-
Collaborate with human agents to co-pilot financial decisions, provide recommendations, execute tasks, and learn from feedback.
In essence, Agentic AI transforms banking from reactive automation to proactive intelligence—empowering banks to deliver hyper-personalized services, faster loan approvals, and intelligent financial advisory at scale.
Traditional Way of Banking and Financial Services
Traditional banking laid the foundation for today’s financial systems, but it came with limitations. Here's a quick look at how banking and financial services functioned in their conventional form:
-
Branch-Centric Operations: Banking was primarily done in person at physical branches. Customers had to visit for services like account management, transactions, and loans, leading to delays and inconvenience.
-
Limited Technology Integration: Legacy systems with minimal automation were used. Data was stored in silos, making operations slow and inefficient.
-
Slow Customer Service: Most processes were manual, resulting in long wait times for approvals, KYC, and issue resolution. No real-time or 24/7 support was available.
-
Standardized Product Offerings: Banks offered basic, non-personalized products like savings accounts and loans. There was little focus on customer needs or preferences.
-
Manual Risk & Compliance: Risk management and compliance relied on paper-based processes. Fraud detection was reactive, not real-time.
-
Limited Reach & Accessibility: Services were focused in cities, with rural areas underserved. People with disabilities or limited literacy faced difficulties accessing banking.
While traditional banking established trust and structure, it lacked the speed, personalization, and accessibility modern customers expect. The evolution of digital and intelligent financial services addresses these gaps and sets the stage for future-ready banking.
Impact on Customers Due to Traditional Banking and Financial Services
Traditional banking shaped early financial experiences but often posed challenges for customers.
Below are the key impacts customers face due to conventional banking methods:
-
Inconvenience and Time-Consuming Processes: Customers had to visit physical branches during limited hours for even simple tasks. This made banking difficult for working professionals, rural residents, and those with limited mobility.
-
Delayed Services and Approvals: Manual processing of transactions, KYC, and loan applications led to long wait times. Customers experienced frustration due to a lack of real-time services.
-
Lack of Personalization: Bank offerings were generic and not tailored to individual needs. Customers receive the same products and services regardless of their financial goals or behaviour.
-
Limited Access to Financial Services: People in remote or underserved regions had minimal access to banks. Additionally, individuals with low literacy, the elderly, or disabled faced barriers in navigating traditional systems.
-
Poor Customer Support Experience: Support was available only during business hours, mostly via in-person visits or phone calls. There were no chatbots, self-service apps, or 24/7 assistance.
-
Low Financial Inclusion: Traditional banking often excluded individuals without formal documentation or consistent income, making it harder for them to access credit or open accounts.
These limitations created a clear divide between customer expectations and actual service delivery. The rise of digital and intelligent banking aims to close this gap by offering convenience, personalization, and inclusivity.
Key Use Cases of Agentic AI in Banking
Fig 1: Use Cases of Agentic AI in Banking
1. Personalized Recommendations
Description: Tailored financial advice based on individual behaviours and goals.
Opportunity: Enhances customer satisfaction and engagement but requires attention to privacy, bias, and explainability.
Agentic AI Solutions:
-
Advanced data analysis to understand spending habits and goals
-
NLP to analyze conversations and interactions
-
Predictive modelling to anticipate future financial needs
-
Dynamic customer profiles that update in real-time
-
Built-in compliance checks and transparent explanations
2. Real-time Monitoring
Description: Continuous surveillance of financial transactions and user activity.
Opportunity: It boosts fraud detection and user trust but demands strong regulatory and privacy governance.
Agentic AI Solutions:
-
Anomaly detection to flag suspicious behaviour
-
Predictive analysis to identify potential risks early
-
Behavioural analytics for customer segmentation
-
Cybersecurity integration for threat mitigation
-
Automated regulatory compliance
3. Predicting Credit Risk
Description: Assessing borrower risk for better credit decisions.
Opportunity: Accelerates decision-making while reducing human bias.
Agentic AI Solutions:
-
Analysis of financial behaviour and patterns
-
Behavioral scoring based on transaction history
-
Predictive models to forecast default risks
-
Transparent credit decisions for regulators and customers
4. Analyzing Market Trends
Description: Monitoring and forecasting shifts in the financial landscape.
Opportunity: Simplifies complex data analysis for faster decision-making.
Agentic AI Solutions:
-
Real-time data streaming for prompt responses
-
Predictive analytics based on historical patterns
-
Sentiment analysis from media and social channels
-
Continuous monitoring of global financial indicators
5. Automated Loan Approvals
Description: Streamlined credit evaluation and disbursement processes.
Opportunity: Speeds up approvals while maintaining accuracy and fairness.
Agentic AI Solutions:
-
Document automation and intelligent form-filling
-
Chatbots for customer communication and status updates
-
Fraud detection in loan applications
-
Risk scenario simulations for robust decisions
6. Pitchbook Creation
Description: Dynamic presentations for client engagement and investment proposals.
Opportunity: Saves time by automating research and formatting.
Agentic AI Solutions:
-
Multi-source data aggregation for market insights
-
Summarization of key financial metrics and trends
-
Personalization of content for different client segments
7. Customer Onboarding
Description: Simplifying the process of welcoming new customers.
Opportunity: Converts a traditionally manual, time-consuming task into a seamless experience.
Agentic AI Solutions:
-
OCR for extracting data from KYC documents
-
Intelligent validation of customer inputs
-
Automated integration into CRM and banking systems
-
Real-time regulatory checks
8. KYC and Anti-Money Laundering (AML)
Description: Ensuring legal compliance and preventing financial crime.
Opportunity: Enhances monitoring and reduces regulatory risk.
Agentic AI Solutions:
-
AI-driven detection of suspicious transactions
-
Pattern recognition for identifying anomalies
-
Monitoring of high-risk profiles
-
Automated SAR (Suspicious Activity Report) generation
9. Automatic Report Generation
Description: Creating regulatory and operational reports with minimal human input.
Opportunity: Increases efficiency, accuracy, and audit-readiness.
Agentic AI Solutions:
-
Natural Language Generation for readable reports
-
Data extraction and consolidation from multiple systems
-
Real-time compliance validation
-
Report scheduling and archival automation
10. Daily Sales Reconciliation (DSR)
Description: Aligning daily sales records with bank statements.
Agentic AI Solutions:
-
Automatic extraction and matching of transaction data
-
Real-time discrepancy alerts and resolutions
-
Reduction in manual errors and processing time
11. Bank Reconciliation (BRS)
Description: Ensuring financial records match bank accounts.
Agentic AI Solutions:
-
Automated comparison of financial reports with bank data
-
Handling of checks and credit card transactions
-
Increased transparency and accuracy
12. Accounts Payable and Receivable Automation
Description: Automating invoice approvals, vendor payments, and cash collection for improved financial workflow.
Agentic AI Solutions:
-
Scans and extracts data that matches purchase orders.
-
Automates payment approvals for timely processing.
-
Sends automated overdue payment alerts.
-
Monitors payables and receivables continuously.
-
Ensures accurate financial tracking and reduces delays.
13. Tax Compliance Automation
Description: Simplifying tax reporting and filing processes.
Agentic AI Solutions:
-
Auto-extraction of tax-relevant financial data
-
Timely and accurate tax return generation
-
Audit support and compliance validation
14. Fraud Detection and Prevention
Description: Detecting and preventing financial fraud in real-time.
Agentic AI Solutions:
-
Continuous transaction monitoring
-
Pattern-based detection of anomalies
-
Instant alerts and response mechanisms
15. Financial Reporting Automation
Description: Automating the generation of financial reports for internal and external stakeholders.
Agentic AI Solutions:
-
Data aggregation from multiple departments
-
Real-time dashboard updates
-
Generation of regulatory-compliant financial statement
Successful Implementation of AI Agents in Banking and Financial Services
Zurich Insurance: Global insurer Zurich has implemented AI and freed up to 40% of its commercial underwriter process. This has allowed them to focus on high-value-added tasks and devote more time to complex policies. Zurich related that its pilot program realized a 50% cost reduction, motivating it to expand its implementation further. OCBC Bank (Singapore): OCBC Bank, a prominent Singaporean bank, reduced the time required to re-price home loans from 45 minutes to just 1 minute by deploying intelligent automation. The AI not only re-prices the loans but also checks customers' eligibility, recommends options, and drafts the necessary recommendation emails, significantly improving efficiency and reducing human intervention Sumitomo Mitsui Banking Corporation (Japan): Sumitomo Mitsui, a major Japanese financial institution, leveraged AI to cut out 400,000 hours of manual labor annually. By automating its processes, the institution significantly boosted its operational efficiency, freeing up time for employees to focus on more strategic tasks.
The Future of Agentic AI in Banking
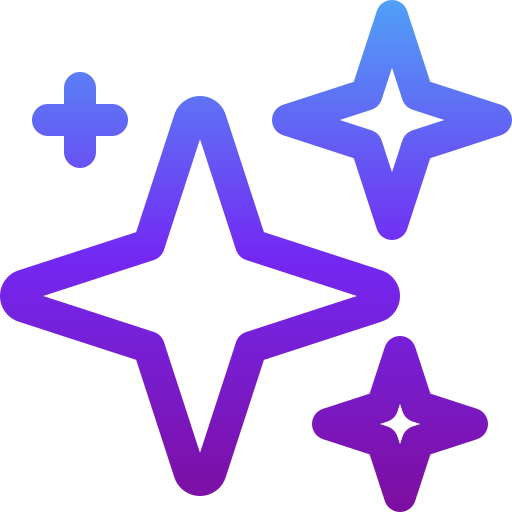
Enhanced Operational Efficiency
Agentic AI will automate repetitive tasks like data extraction and report generation, boosting productivity for early adopters by 22% to 30%. This allows banks to allocate resources more effectively and concentrate on higher-value activities.
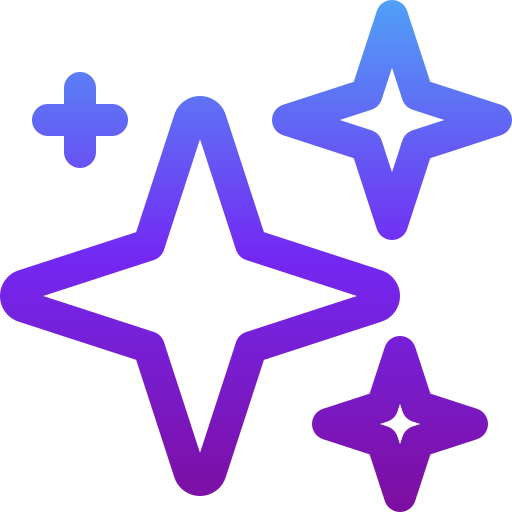
Personalized CX
Financial institutions will utilize agentic AI to deliver tailored services, such as personalized financial advice and customized product recommendations. AI-driven chatbots will also enhance customer support, improving overall satisfaction.
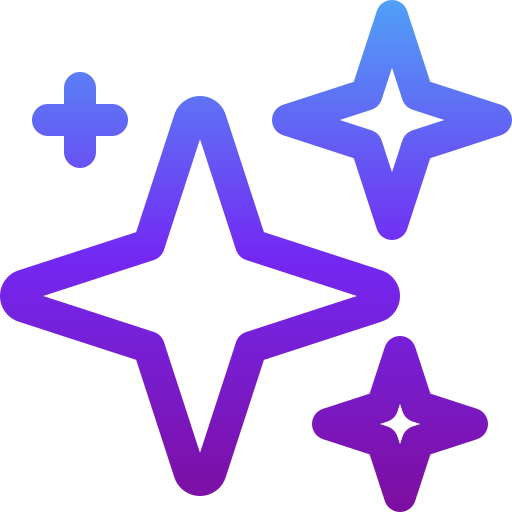
Advanced Risk Management
Agentic AI will enhance risk assessment and fraud detection by analyzing large datasets in real-time. This will enable banks to identify suspicious transactions and evaluate creditworthiness more accurately, reducing financial losses and ensuring regulatory compliance.
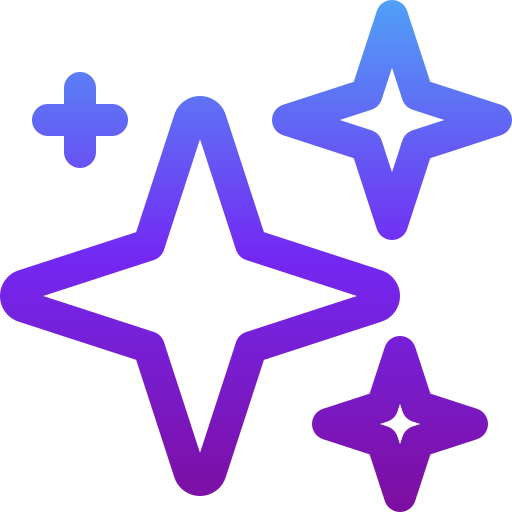
Revenue Growth and Innovation
The integration of agentic AI is expected to add $200 billion to $400 billion in value to the banking sector by 2030. This technology will drive efficiency and foster innovation in product development and service delivery, helping banks remain competitive in a changing market.
Benefits of Agentic AI in Finance and Banking
Fig 2: Benefits of Agentic AI in Finance And Banking
-
Personalized Customer Experience: By analyzing customer behaviour, preferences, and transaction history, banks can offer highly tailored financial services. Real-time, relevant recommendations improve customer satisfaction and foster stronger, more loyal relationships.
-
Operational Efficiency: Routine tasks like onboarding, reconciliations, and financial reporting can be automated, reducing the time and effort spent on manual processes. This allows teams to focus on high-value work and ensures smoother operations overall.
-
Real-time Fraud Detection: Continuous monitoring of transactions and user behaviour helps instantly identify anomalies and potential threats. Early detection enables quick action, minimizing financial losses and boosting customer security.
-
Smarter Decision-Making: Access to large datasets provides valuable insights that drive informed decisions on credit scoring, risk management, and investments. This ensures faster, more accurate financial outcomes and helps reduce biases in decision-making.
-
Regulatory Compliance: Automating compliance checks and report generation ensures financial institutions stay aligned with evolving regulations. It streamlines reporting processes and minimizes the risk of fines or penalties for non-compliance.
-
Cost Optimization: Automation reduces reliance on manual labour, cuts operational costs, and improves processing efficiency. This results in faster decision-making, better resource utilization, and higher ROI across financial operations.
Challenges to Agentic AI Adoption
Despite its numerous benefits, the adoption of agentic AI in banking faces several challenges:
-
Data Privacy and Security Concerns: AI systems require vast amounts of sensitive customer data, making it crucial to ensure robust data protection measures and compliance with regulations like GDPR.
-
Regulatory Compliance and Ethical Considerations: Navigating the complex landscape of financial regulations and ensuring AI decisions are transparent, unbiased, and ethical can be challenging and time-consuming.
-
Integration with Legacy Systems: Many financial institutions rely on outdated systems that are difficult to integrate with new AI technologies, leading to high integration costs and potential disruptions.
-
Talent and Expertise Shortage: The shortage of skilled professionals in AI, machine learning, and data analytics challenges the effective deployment and maintenance of AI systems in finance.
-
Bias in AI Algorithms: AI models can unintentionally perpetuate biases if the training data is not diverse or representative, which could lead to unfair decisions and reputational risks.
Next Steps towards Agentic AI in Banking
Talk to our experts about implementing Agentic AI systems in banking. Discover how financial institutions and departments leverage AI-driven workflows and Decision Intelligence to enhance decision-making. Utilize Agentic AI to automate customer interactions, streamline loan processing, and optimize fraud detection, improving efficiency and customer satisfaction.