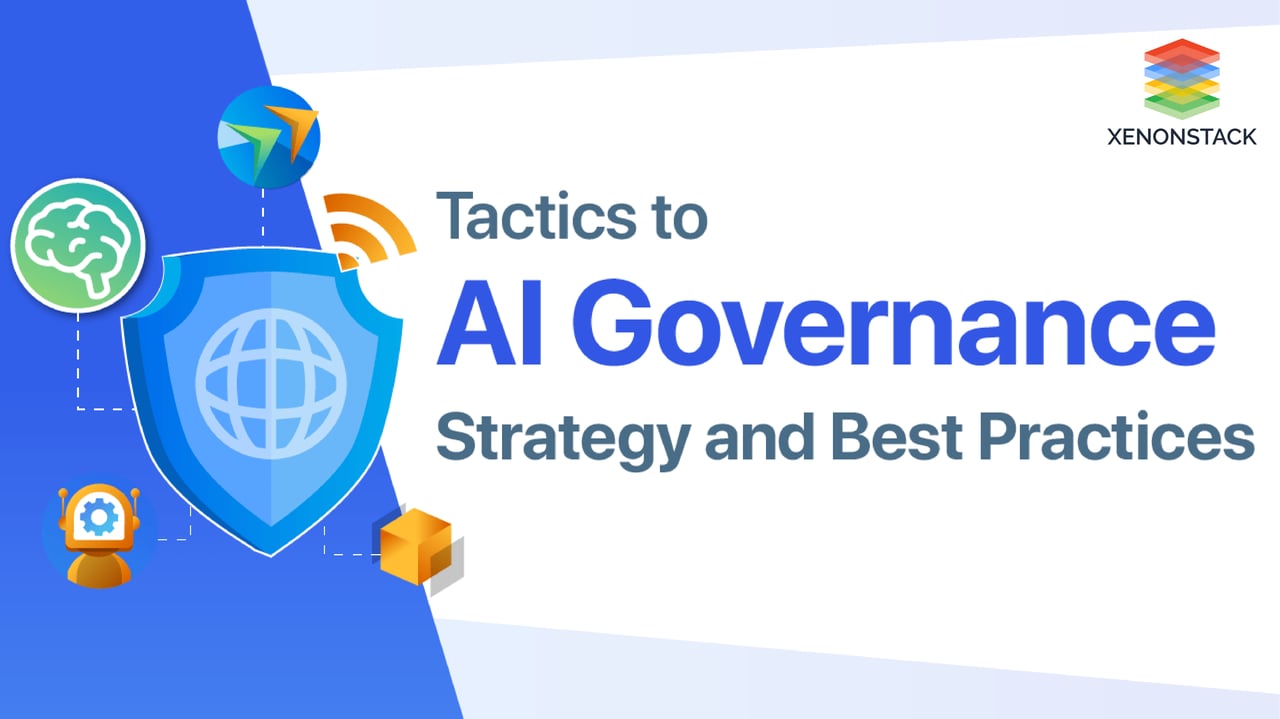
Overview of AI Governance Strategy
To drive business growth, the ability to leverage AI is a compelling proposition across industries today. It uses data to make decisions. According to a report, approximately three-quarters of businesses consider AI for their success due to the continuous advancement of AI in making decisions.
Due to increasing demands and the rush to design, develop and deploy AI applications, many organizations fail to challenge AI governance due to consideration. However, it is becoming clear that failing to address Artificial Intelligence governance properly can have far-reaching consequences, such as failing to address data protection concerns. It can cause significant financial harm, damage a company's image, and even cross ethical boundaries. Some see governance as bureaucracy or an obstacle; it may be discouraging.
Artificial Intelligence can help create machines to perform specific tasks that normally require human intelligence. Click to explore our, AI Adoption Challenges and Solutions
What is Artificial Intelligence Governance?
AI Governance has three key areas:
AI model Definition
It must clearly define the objective of the AI model. Such as what an organization wants to achieve from the model and is the AI model capable of achieving it. Is it explainable to others? These things help in troubleshooting.
AI model Management
It is important to track and develop model catalogs to ensure the right model for the right objective or requirement. This catalog will help ensure the work of models that it can do, limitations that it cannot do, the purpose of models, and modification details. It also tracks
- Requirements for training data
- Requirements for deployment
- Key metrics that need to be achieved
- Continuously monitoring the model for model drift
Data Governance
The success of Artificial Intelligence models depends on high data quality; therefore, effective data governance is essential for their success. It helps to answer some questions:
- What type of data is it?
- What is the data source and lineage?
- How can data be transformed or modified?
- Who modified the data last time, and who can modify it?
- Does data contain any sensitive information?
- Does it comply with privacy regulations?
What are the Challenges of AI Governance?
Companies are just focusing on the process and decision by giving data; they are not bothering with the data quality. Bad data led to bad outcomes. Therefore companies utilizing bad data to make decisions are getting bad decisions or results. Therefore, it must have good training data with good management techniques for its models to have correct decisions. Therefore Artificial Intelligence governance is a necessity, not an option. Having good data and saving it from misuse is required to implement a successful AI governance strategy.
According to the report of O’Reilly, only slightly more than 20% of organizations implemented formal AI governance. They think that implementing AI governance is an option, not an essential one. But we have to understand that it is not an option, it is a necessity.
Discover more about Privacy-Preserving AI with a Case-Study
Why do we need a Successful Artificial Intelligence Governance Strategy?
In the last topic, we discuss that AI governance is a necessity, not an option. Now Let’s discuss why we need this. It not only keeps the company safe but also brings the data standards into implementation.
- Save Money: According to Gartner, recent research shows that organizations believe that approximately $15 million per year of losses is due to poor data quality. The cost due to a security breach is also very huge. According to the IBM report, $3.92 million is an average cost of a data breach. A robust governance approach can save a lot of money by handling data quality and security.
- Improve Trust: AI Governance (Artificial Intelligence governance), properly implemented, can improve trust in data and systems at all levels of an organization. It allows employees to be more confident in the decisions systems are making with data. It can also improve trust in the analysis and models produced by data scientists, with greater accuracy resulting from improved data quality.
- Reduce Risk: Ensuring ethics, management, and security reduces company reputation risk from data breached unethical responses issues.
What are the approaches for a successful Artificial Intelligence Governance strategy?
5 Keys to defining a successful AI Governance strategy:
- A top-down and bottom-up Strategy: Every AI Governance strategy needs executive sponsorship. It Is required to have strong leadership support To improve data quality, overall security, and management. Parallelly individual team has to take responsibility for the data, model, and tasks they manage. There should be continuous integration and an ownership culture of data issues. This bottom-up strategy can only be achieved by top-down communication and recognition.
- Quality is the Heart: In most organizations, data products are produced by data scientists and business intelligence teams. They have not had the same commitment to quality as traditional software development through movements such as software craftsman and extreme programming. But this is no longer the case. Data products should have high quality, using code review, testing, CI/CD.
- Balance Between Governance and Enablement: Governance should support innovation rather than become a blocker for innovation.
- Model Management: Model management becomes a key factor in Artificial Intelligence Governance. A model can degrade over time to resolve this issue, it is required to check the performance and model drift timely. Continuous monitoring, model refreshes, and testing need to ensure model performance meets the business expectations.
- Transparency and Responsible AI: The model's decision directly impacts human lives; therefore, it is necessary to track how the model makes decisions. Whether the decisions that it makes are correct or not. Therefore explanation is required to spot issues like bias and mitigate them. It is impossible to track these things in an opaque model; therefore, an approach is required to have transparent AI applications.
When data is processed, it is collected and transformed into useable information. Explore here the Architecture of Data Processing
What are the Best Practices of Artificial Intelligence Governance?
Some key steps of AI Governance:
- First, it is vital to prioritize the goals and take on governance tasks appropriate for the sophistication of AI projects.
- By defining goals, we can track whether a model performs as expected or not. Thus it also ensures fairness in the model by eliminating bias and determining the issues that could impact success.
- Ensure standards and best practices of Artificial Intelligence governance by reviewing them periodically. Track key metrics that need to be measured, a process to track data, and monitor overall model performance.
Conclusion
Practitioners understand that proactive governance prevents them from bad decisions and spurs creativity, optimizes capital, and aids in realizing project or company benefits when performed correctly. Moreover, as AI Governance continues to evolve, companies may need to develop basic AI governance capabilities to get the most from their AI projects.
- Discover more about Real-Life Ethical Issues of Artificial Intelligence
- How to enable Artificial Intelligence (AI) Solutions on Edge