Overview of Continuous Delivery
In this post, We’ll share the process how you can Develop and Deploy Python Application using Docker and Kubernetes and adopt DevOps in existing Python Applications. Continuous delivery is a branch of continuous integration. It concentrates on automating the software delivery process so that teams can quickly and confidently deploy their code to production at any point.CI concentrates on automating the software delivery process so that teams can quickly and confidently deploy their code to production at any point. Click to explore about, Continuous Delivery Pipeline with Jenkins
Prerequisites are mentioned below
To follow this guide you need- Kubernetes
- Kubectl
- Shared Persistent Storage - Option are GlusterFS, Ceph FS, AWS EBS, AzureDisk etc.
- Python Application Source Code
- Dockerfile
- Container-Registry
Kubernetes is an open source platform that automates container operations, and Minikube is best for testing kubernetes in a local environment.
Kubectl is command line interface to manage kubernetes cluster either remotely or locally. Shared Persistent Storage is permanent storage that we attach to the kubernetes container. We will be using cephfs as a persistent data store for kubernetes container applications.
Application Source Code is the source code that we want to run inside a kubernetes container. Dockerfile contains all the actions that are performed to build python application. The Registry is an online image store for container images. Below mentioned options are few most popular registries.
- Private Docker Hub
- AWS ECR
- Docker Store
- Google Container Registry
Building Dockerfile for Python applications
The Below mentioned code is sample docker file for Python applications. In which we are using python 2.7 development environment.
FROM python: 2.7
MAINTAINER XenonStack
# Creating Application Source Code Directory
RUN mkdir - p / usr / src / app
# Setting Home Directory
for containers
WORKDIR / usr / src / app
# Installing python dependencies
COPY requirements.txt / usr / src / app /
RUN pip install--no - cache - dir - r requirements.txt
# Copying src code to Container
COPY. / usr / src / app
# Application Environment variables
ENV APP_ENV development
# Exposing Ports
EXPOSE 5035
# Setting Persistent data
VOLUME["/app-data"]
# Running Python Application
CMD["python", "wsgi.py"]
The Below mentioned command will build your application container image.
Building Python Docker Image
The Below mentioned command will build your application container image.
$ docker build - t < name of your python application > : < version of application > .
Publishing Container Image
To publish Python container image, we can use different private/public cloud repository like Docker Hub, AWS ECR, Google Container Registry, Private Docker Registry.- Adding Container Registry to Docker Daemon
$ docker version
Client:
Version: 17.03 .1 - ce
API version: 1.27
Go version: go1 .7 .5
Git commit: c6d412e
Built: Mon Mar 27 17: 14: 09 2017
OS / Arch: linux / amd64(Ubuntu 16.04)
Now we need to Create a “daemon.json” in below-mentioned location
$ sudo nano / etc / docker / daemon.json
And add the following content to it.
{
"insecure-registries": ["<name of="" private="" registry="" your="">"]
}
Now Run the following commands to reload systemctl and restart docker daemon.
$ sudo systemctl daemon - reload
$ sudo service docker restart
To verify that your container registry is added to local docker daemon, use the below-mentioned steps.
$ docker info
In output of above, you get your container registry like this
Insecure Registries:
<your container="" name="" registry="">
127.0.0.0/8
- Pushing container Images to Registry
#!/bin/bash
pip install --upgrade --user awscli
mkdir -p ~/.aws && chmod 755 ~/.aws
cat << EOF > ~/.aws/credentials
[default]
aws_access_key_id = XXXXXX
aws_secret_access_key = XXXXXX
EOF
cat << EOF > ~/.aws/config
[default]
output = json
region = XXXXX
EOF
chmod 600 ~/.aws/credentials
ecr-login=$(aws ecr get-login --region XXXXX)
$ecr-login
Now we need to retag python application image and push them to docker hub container registry. To Retag application container image
$ docker tag < name of your application > : < version of your application > < aws ecr repository link > /<name application="" of="" your="">:<version application="" of="" your="">
To Push application container Images
$ docker push < aws ecr repository link > /<name application="" of="" your="">:<version application="" of="" your="">
Configure Persistent Volume (optional)
Persistent Volume is only required if your application has to save some data other than a database like documents, images, video etc. then we need to use the persistent volume that kubernetes support like was AWS EBC, CephFS, GlusterFS, Azure Disk, NFS etc. Today I will be using cephfs(rbd) for persistent data to kubernetes containers. We need to create two files named persistent-volume.yml and persistent-volume-claim.yml- Persistent Volume
-- -
apiVersion: v1
kind: PersistentVolume
metadata:
name: app - disk1
namespace: < namespace of Kubernetes >
spec:
capacity:
storage: 50 Gi
accessModes:
-ReadWriteMany
cephfs:
monitors:
-"172.16.0.34:6789"
user: admin
secretRef:
name: ceph - secret
readOnly: false
- Persistent Volume Claim
-- -
apiVersion: v1
kind: PersistentVolumeClaim
metadata:
name: appclaim1
namespace: < namespace of Kubernetes >
spec:
accessModes:
-ReadWriteMany
resources:
requests:
storage: 10 Gi
- Adding Claims to Kubernetes
$ kubectl create - f persistent - volume.yml
$ kubectl create - f persistent - volume - claim.yml
Creating Deployment Files for Kubernetes
Deploying application on kubernetes with ease using deployment and service files either in JSON or YAML format.- Deployment File
apiVersion: extensions / v1beta1
kind: Deployment
metadata:
name: < name of application >
namespace: < namespace of Kubernetes >
spec:
replicas: 1
template:
metadata:
labels:
k8s - app: < name of application >
spec:
containers:
-name: < name of application >
image: < image name > : < version tag >
imagePullPolicy: "IfNotPresent"
ports:
-containerPort: 5035
volumeMounts:
-mountPath: /app-data
name: < name of application >
volumes:
-name: < name of application >
persistentVolumeClaim:
claimName: appclaim1
- Service File
apiVersion: v1
kind: Service
metadata:
labels:
k8s - app: < name of application >
name: < name of application >
namespace: < namespace of Kubernetes >
spec:
type: NodePort
ports:
-port: 5035
selector:
k8s - app: < name of application >
Running Python Application on Kubernetes
Python Container Application can be deployed either by kubernetes Dashboard or Kubectl (Command line). I`m using the command line that you can use in production kubernetes cluster.$ kubectl create - f < name of application > .deployment.yml
$ kubectl create - f < name of application > .service.yml
Now we have successfully deployed Python Application on Kubernetes.
Verifying Dockerfile for Python Applications
We can verify application deployment either by using Kubectl or Kubernetes Dashboard. Below mentioned command would show you running pods of your application with status running/terminated/stop/created.
$ kubectl get po--namespace = < namespace of kubernetes > | grep < application name >
Result of above command 
Testing Dockerfile for Python Applications
Get the External Node Port using the below mentioned command. External Node Port is in the range from 30000 to 65000.
$ kubectl get svc--namespace = < namespace of kubernetes > | grep < application name >
Launch web Browser and open any of the below-mentioned URLs.
- http://<kubernetes master ip address >: <application service port number>
- http://<cluster ip address >: <application port number>
Troubleshooting Dockerfile
- Check Status of Pods.
- Check Logs of Pods/Containers.
- Check Service Port Status.
- Check requirements/dependencies of application.
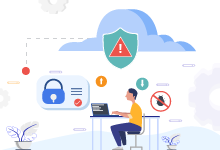
Our solutions cater to diverse industries with a focus on serving ever-changing marketing needs. Click here for our DevOps Consulting Services and Solutions
How can XenonStack Help You?
Our DevOps Consulting Services provides DevOps Assessment and Audit of your existing Infrastructure, Development Environment and Integration. We provide End-To-End Infrastructure Automation, Continuous Integration, Continuous Deployment with automated Testing and Build Process. Our DevOps Solutions enables Continuous Delivery Pipeline on Microservices and Serverless Computing on Docker, Kubernetes, Hybrid and Public Cloud. Our DevOps Professional Services includes -- Single Click Deployment
- Continuous Integration and Continuous Deployment
- Support Microservices and Serverless Computing - Docker and Kubernetes
- Deploy On-Premises, Public or Hybrid Cloud
- Learn more about "Azure DevOps Pipeline for Laravel Php"
- Read more about "Continuous Delivery for Scala Applications"